AI enables early response to damp and mould risk at Wolverhampton Homes

Sam Dugmore from Wolverhampton Homes explains how AI has helped predict and prevent issues with damp and mould and improve resident satisfaction.
The government’s pledge to deliver 1.5 million new homes by 2028 is a step forward for many families in need of affordable housing. However, as demand grows for social housing, landlords will face increasing pressure to not just build more homes, but to ensure they are healthy, safe and habitable.
With Awaab’s Law coming into effect from October 2025, social housing providers will need to act swiftly to meet stricter standards on tackling issues such as damp and mould, which can put vulnerable residents’ health at risk and damage properties.
At Wolverhampton Homes, we’ve been looking at how AI can be used to help us meet this challenge as our housing stock continues to grow.
Taking action
We recently partnered with NEC Housing to explore how AI technology could help predict the risk of damp and mould risk in housing.
Data from thousands of our homes was analysed, including details such as the condition of properties, maintenance history and whether complaints of damp and mould had been received before. Environmental factors were taken into consideration too, such as local flooding and weather patterns.
The results of the initiative were striking – we found AI correctly identified properties at risk of damp and mould with 98% accuracy.
This showed us that AI and machine learning could be used to help social landlords to address damp and mould more effectively in three key ways.
1. Spot problems before they get worse
Issues such as damp and mould are typically dealt with after a report is received from the tenant. AI could change that by allowing housing providers to flag homes most at risk before problems even appear.
With information such as the age and location of properties, humidity levels and whether extractor fans are working in the home, it is possible to use AI technology to prioritise repairs or schedule inspections for those properties which are flagged as most at risk.
2. Act faster to address issues
The quality of information is critical to understand which households need to be prioritised to prevent or rectify damp and mould. We found that when AI was tested with incomplete information/data, the accuracy of predictions decreased from 98% to 70%. These findings have further cemented and confirmed Wolverhampton Homes’ commitment to ensuring that we consistently and update our housing management system, with the most up-to-date information we have to support data-decision-making from this model.
We want our staff to be able to keep records up to date easily. If one of our housing officers finds out a resident has developed a health issue, or notices a problem with condensation during a visit, for example, they can immediately update the information in our NEC Housing system.
This will automatically adjust the damp and mould risk level and move the household up the priority list for repairs, helping us to keep our homes safe.
3. Planning for the future
Following the successful pilot of the DMC Predictability Model and understanding its potential benefits and usage within Wolverhampton Homes, our vision is to use this AI to help us to organise day-to-day repairs more efficiently, focusing on priority cases and our most vulnerable tenants. It will also allow us to develop a long-term strategy to address damp and mould.
We can identify patterns and trends across multiple properties or local areas to see which types of homes need better ventilation, insulation or maintenance work and target improvements where they are most needed. For example, if AI finds that homes built in the 1960s in high-rainfall areas are more likely to have structural issues, this insight can guide retrofit projects to focus on long-term improvements for these older properties.
A new way forward
AI has the potential to transform the way social housing providers manage properties, not only by preventing damp and mould but by improving energy efficiency, reducing long-term costs, and enhancing resident satisfaction.
The challenges ahead require the sector to explore new and different ways to improve their homes and the experience of residents. AI could be a valuable addition to the social housing toolbox.
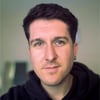
By Sam Dugmore
Sam is the Systems Development and Support Manager at Wolverhampton Homes.Also Read
- Spatial analysis helps locate new women’s and girls’ health hubs in south east London
- Where are we at and where are we going? Cloudera leaders on integrating AI into successful government transformation
- "The amount of data government has is truly extraordinary," Akamai's Richard Meeus on public sector data sharing
- New law will see government data being harnessed to slash bureaucracy and increase efficiency